A Tale of Two Sentiments: Text Sentiment vs. Deal Sentiment
You sharpen competitive edges wherever you can.
Sales leaders are always looking for ways to leverage the power of AI and machine language intelligence to boost team productivity.
To this end: AI injects tools with language analysis, determining customer sentiment in rep email exchanges, online chats, and call transcripts.
Then, text sentiment detects positive emotion when a customer is highly engaged.
AI needs a ramp
But just as a new sales rep requires onboarding and training, a typical machine learning model needs time to understand the language of your sales motion.
It has to be able to analyze your organization’s wins and losses—and the language used throughout those transactions—over a period of months or even years.
Which means that text sentiment isn’t the same thing as deal sentiment. And an off-the-shelf sentiment scorer is never the best barometer of deal progression.
Generic sentiment model: Captures emotion
Up for a simple analysis? There are lots of off-the-shelf sentiment models you can download, utilize through API services, or bake into sales productivity tools.
And it’s not too hard to get a sentiment metric for a body of email, chat, or call transcript text.
For example, you can score sales emails using a common sentiment model. But for the sentiment to be useful, it should indicate deal progress. And it doesn’t.
When you plot sentiment score against deal win rate (see “Sentiment” vs. Win chart, below), there’s no meaningful correlation.
Emails that score high in sentiment are no more likely to win deals than emails that score low.
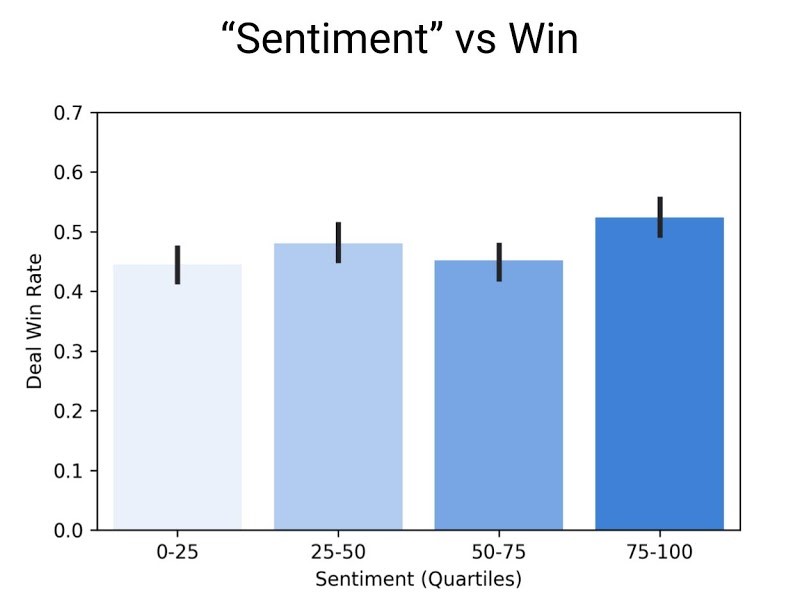
But history tells you deals are won when customers are engaged and positive about your product.
So what’s up?
Deal sentiment model: Captures progression
Let’s repeat the same analysis, but this time, using a different sentiment model: a deal sentiment model.
This model, built by the Data Science Team at SetSail, analyzes the same collection of emails. Unlike the generic sentiment model above, this deal sentiment model is specifically tuned to historical wins vs. losses data, and the languages used within.
Right away, you can see that the deal sentiment score is much more effective in capturing deal progression (see “Deal Sentiment” vs. Win chart, below).
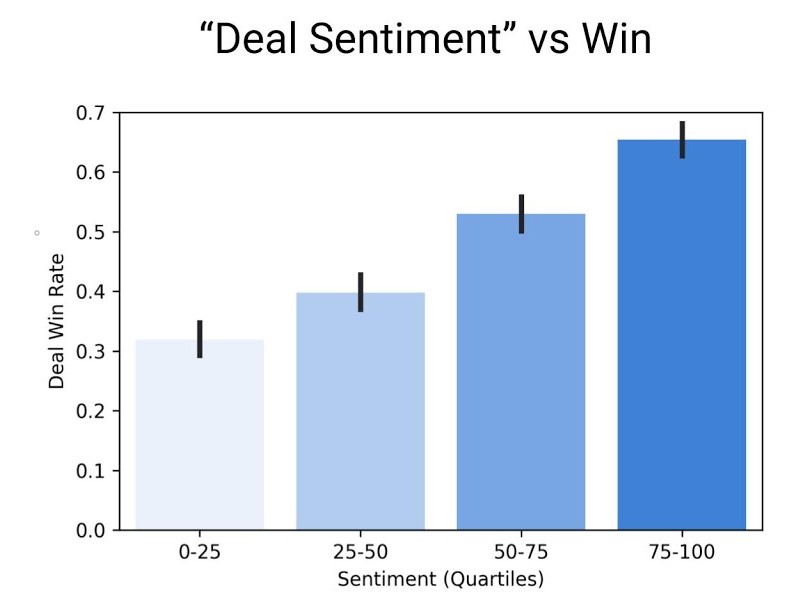
Emails in the top quartile of deal sentiment have more than twice the win rate as emails in the bottom quartile.
How a sentiment model ‘thinks’
Why do the two models perform so differently? Let’s look under the hood at how each “thinks.”
Conventional, or text sentiment models are trained on data that’s irrelevant to the sales process. They’re also typically built to capture emotional sentiment.
Study their behaviors by comparing client sentences that are scored high versus low in emotional sentiment:
Messages scored high in “emotional sentiment”:
- “Sure, send me your calendar link please :)”
- “Excellent. We are on it.”
- “That’s helpful, thanks!”
Messages scored low in “emotional sentiment”:
- “Sorry, I’m running a few minutes behind.”
- “My apologies for the delayed response.”
- “Unfortunately I may need some help with implementation.”
In comparison, here are examples of prospect communications from the deal sentiment model:
Messages scored high in “deal sentiment”:
- “Can you add the bundle pricing to the contract?”
- “I'll sync up with our executive sponsor today.”
- “Confirming that we’ve just signed.”
Messages scored low in “deal sentiment”:
- “I haven't looked into it since we last chatted.”
- “This is not a priority for us right now.”
- “This is definitely interesting, but outside of our budget. We would be happy to reconsider in the future.”
Emotional neutrality
Granted, the above are just a few examples of language used to represent scoring in each model.
But they do help us get a feel for why emotional sentiment isn’t a great indicator of deal progression.
Many professional exchanges in a sales process are emotionally neutral. So using an off-the-shelf sentiment model like a Black Box can give you misleading metrics on how well a deal is going.
In contrast, a sentiment model specifically trained on your organization’s past wins and losses gives you a more accurate measure of deal sentiment.
This type of model learns from the language specific to your sales motion, identifying words, terms, and phrases that indicate true deal movement.
Language as leverage
While machine intelligence is making exciting inroads into language, be thoughtful in leveraging its power for sales productivity.
Emotional sentiment isn’t the answer. Deal sentiment is.
With the SetSail incentive platform, you can reward your reps in the moment as they get positive deal-progression responses from prospects and customers.
The platform builds language models specific to your company’s sales motion. Rather than rewarding reps for positive emotional sentiment, SetSail continuously rewards them for positive deal sentiment.
That can make the difference between a win and a loss.
Ready to see SetSail in action? Request a demo here.