When Machine Learning Meets Human Nature
Machine Learning (ML) is now widely used in the enterprise. 79% of companies are already employing some level of ML to improve their users’ experiences. Whether users are aware of it or not, most products we use have certain elements of ML embedded within. It might be automation, or prediction, or text and image processing. In many use cases, intelligent algorithms are doing chores for us in the background, like a silent worker. When they work well, we don't even feel their presence.
But our aspiration for ML is a lot bigger. We want intelligent algorithms to also make us, the humans, effectively more intelligent. We want them to help us operate like superhumans. Much like how our knowledge is vastly expanded when armed with search engines.
There is one way for such aspiration to materialize. But first, we need to partner ML with another fascinating scientific discipline.
Machine Learning as a Factory of Good Advice
What is machine learning extremely good at? Pattern recognition. Given lots of historical data, ML can extract patterns that are correlated with good vs bad outcomes. Then we can synthesize the results into suggestions and recommendations. In a way then, ML is a scalable and automated factory that churns out "Good Advice".
But there are some caveats. First, ML's advice is only as good as the data fed into it. If there are data issues like coverage or bias, then the quality of the advice will suffer. For example, if we don't have data on shaking hands, then we can't expect ML to tell us that shaking hands with new customers is good for eventually closing deals.
Machine learning is a scientific instrument, and like other scientific instruments such as a microscope or a telescope, ML also has "resolutions". Microscopes and telescopes help us see smaller and further, but there's a limit. We can improve the resolution by innovating on the design of such instruments, just like we can improve ML's advice-giving capability by supplying better data. But for the algorithms currently being deployed, we need to be aware of the existing data state, and correspondingly, the resolution limit.
Another caveat is less about ML and more about us. While we can have ML generate many many "Good Advice", we humans are just famous for not taking them. So famous, in fact, that there are quotes, proverbs, and popular song lyrics lamenting how people are just not taking good advice.
Pairing Machine Learning with Behavioral Economics
Let's now talk about a completely different discipline, behavioral economics. This fascinating area of research is unveiling many mechanisms of human decision-making. We will just very superficially touch on one subarea: the science of habit formation. And we will also very superficially summarize the mechanism of habit formation: action → reward → we feel good → repeat.
This feedback loop can work in our favor if the action is something that, over time, will lead to a good outcome. But how do we know which action is good? A good outcome can take a long time to manifest, like becoming fit, learning a new language, or closing a deal. If we pick the wrong action to reward, it might be ineffective (like knocking on wood, but at least it doesn't hurt right? *knocks on wood*), or worse, we could end up fostering a detrimental habit!
It's almost like if we can combine a "Good Advice" factory with the incentive-driven habit feedback loop, that would be just the perfect recipe. In this sense, ML and Behavioral Economics are a really great fit for each other.
Machine Learning as the Compass; Incentive as the Trail Mix
Imagine you are in a deep forest. You are hiking around, trying to get to the highest point. But you can't see much out of the tall and dense redwoods. This is like the scenario we face in our everyday life. Every moment, there are many decisions we have made. We all want to optimize our actions to reach a good outcome, but it's going to take a while and there's so much about the forest we don't know. With every step, we are worried if what feels like a momentary step up might actually be taking us further away from the goal.
Combining ML and Behavioral Economics is like having a compass and a bag of trail mix with us on this hike. ML lets us know if we are "directionally correct". The algorithm might have limited resolution, and cannot tell us exactly which branch to hop over and to go around which tree, but it can be correct enough to guide us in the right direction. And with every step taken in the right direction, we can keep our spirit up with some snacks. Step by step, we stay on course and motivated, and get to our goal a lot faster (and a lot happier) than frustratingly trying to get out of the maze on our own.
(If you notice that this analogy is also describing the concept of "gradient descent", a foundational algorithm in machine learning and discrete optimization, thank you! There might be some generalizable learning here about optimization, let it be mathematics or human behavior. But yes it's getting maybe too meta.)
Putting It in Practice
In James Clear's "Atomic Habits", the author suggests, in order to create good habits, "... create an environment where doing the right thing is as easy as possible." That's what the combination of ML and incentive can do. ML ensures our small actions are aligned with our big goals. And incentives keep us going to turn the small actions into consistent habits.
Of course, talking is easy, but implementation is much harder. The conceptual framework of ML plus Behavioral Economics might make sense, but actually deploying such a system, admittedly, will require non-trivial technical effort. At SetSail, we apply this ML + Behavior Economics framework for customer-facing teams. Closing deals can be a long and arduous process, like hiking in a deep forest without guidance. Our goal is to equip your team with both a compass and some trail mix, in the form of an effective micro-incentive strategy. No more anxiety-filled aimless wandering. A setback or rejection doesn't totally deflate us anymore. We are more motivated now to get through all the No's before getting to that Yes when we know we are on the right track. By applying ML in a way that takes into account human tendencies, we can make "doing the right thing as easy as possible."
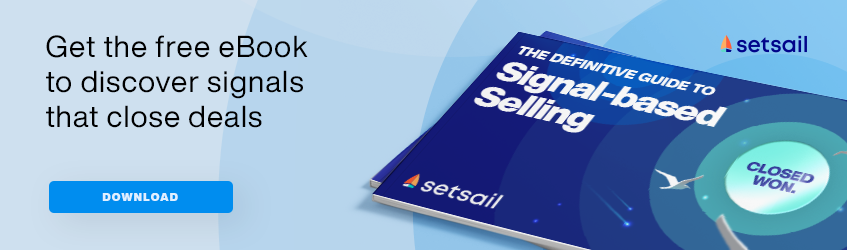